The insurance industry is on the cusp of a major transformation, driven by advancements in artificial intelligence (AI) and machine learning (ML). These technologies are reshaping how insurers assess risk, underwrite policies, handle claims, and interact with customers. This article explores the profound impact of AI and ML on the insurance sector, detailing the significant changes and future prospects.
Introduction to AI and Machine Learning in Insurance
AI and ML refer to computer systems designed to mimic human intelligence and learn from data to improve their performance over time. In the context of insurance, these technologies are used to analyze vast amounts of data to identify patterns, predict outcomes, and automate decision-making processes.
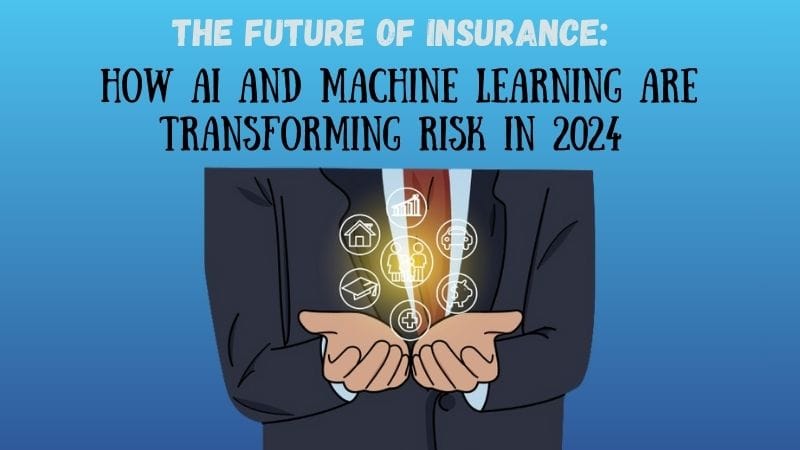
Historical Context
Traditionally, insurance has been a data-driven industry, relying on statistical models and actuarial tables to assess risk and set premiums. However, these methods often involve manual processes and are based on historical data, which may not always accurately predict future risks. The introduction of AI and ML offers a more dynamic and precise approach, leveraging real-time data and advanced algorithms.
Transforming Risk Assessment and Underwriting
Risk assessment and underwriting are at the core of the insurance business. These processes determine the likelihood of a claim being made and the premium to be charged. AI and ML are revolutionizing these areas by providing more accurate and granular insights.
Enhanced Data Utilization
AI and ML enable insurers to analyze a wider range of data sources, including:
- Structured Data: Traditional data points such as age, gender, and health history.
- Unstructured Data: Information from social media, wearable devices, and telematics.
By integrating these diverse data types, insurers can create more comprehensive risk profiles.
Predictive Analytics
ML algorithms can predict future risk based on historical data and emerging trends. For example, predictive models can forecast the likelihood of health issues by analyzing genetic information and lifestyle data. This allows for more personalized underwriting and pricing.
Real-time Risk Monitoring
AI-powered systems can continuously monitor risk factors in real-time. For example, telematics devices in cars provide real-time data on driving behavior, enabling insurers to adjust premiums based on actual driving habits rather than estimates.
Revolutionizing Claims Processing
Claims processing is another area where AI and ML are making significant inroads. The traditional claims process can be slow and prone to errors, but AI and ML offer ways to streamline and improve it.
Automated Claims Management
AI-driven automation can handle routine claims processing tasks, such as:
- Fraud Detection: AI can identify fraudulent claims by detecting anomalies and patterns indicative of fraud. This reduces losses and speeds up legitimate claims.
- Damage Assessment: ML algorithms can analyze images of damaged property or vehicles to estimate repair costs accurately. This accelerates the assessment process and enhances accuracy.
- Customer Communication: AI-powered chatbots can handle customer inquiries and provide updates on claim status, improving customer satisfaction and reducing the workload on human agents.
Faster Settlements
By automating much of the claims process, AI and ML can significantly reduce the time it takes to settle claims. This leads to quicker payouts for policyholders and lower administrative costs for insurers.
Enhancing Customer Experience
AI and ML are not only transforming back-end processes but also enhancing the overall customer experience.
Personalized Customer Interactions
AI-driven analytics can provide personalized recommendations and tailored insurance products based on individual customer profiles. This can range from suggesting the most suitable coverage options to offering wellness programs that help customers reduce their risk.
Improved Accessibility
AI-powered chatbots and virtual assistants make it easier for customers to interact with insurers at any time. These tools can handle a variety of tasks, from answering questions about policy details to helping customers file claims.
Proactive Risk Management
Insurers can use AI and ML to offer proactive risk management advice. For example, an insurer might alert a homeowner to potential flood risks based on weather data and suggest preventative measures to minimize damage.
Operational Efficiency and Cost Reduction
The integration of AI and ML in insurance operations leads to significant efficiency gains and cost savings.
Streamlined Operations
AI can automate routine administrative tasks, such as data entry and policy renewals, freeing up human resources for more complex and value-added activities.
Cost Savings
By improving risk assessment accuracy and reducing fraudulent claims, AI and ML can help insurers lower their overall costs. These savings can be passed on to customers in the form of lower premiums.
Challenges and Considerations
Despite the numerous benefits, the adoption of AI and ML in insurance also presents several challenges and considerations.
Data Privacy and Security
The use of AI and ML involves the collection and analysis of large amounts of personal data. Ensuring the privacy and security of this data is paramount. Insurers must comply with data protection regulations and implement robust cybersecurity measures.
Ethical Concerns
AI and ML algorithms can inadvertently perpetuate biases present in the data they are trained on. This can lead to discriminatory practices in underwriting and claims processing. Insurers must strive to develop fair and transparent algorithms.
Integration with Legacy Systems
Many insurers still rely on legacy IT systems that may not be compatible with modern AI and ML technologies. Integrating these systems can be complex and costly, requiring significant investment in technology upgrades.
Future Trends and Innovations
The future of AI and ML in insurance holds exciting possibilities, with several trends and innovations on the horizon.
Advanced Predictive Modeling
As AI and ML technologies continue to evolve, predictive models will become even more sophisticated. This will enable insurers to anticipate emerging risks, such as those related to climate change or cyber threats, with greater accuracy.
Increased Use of IoT Devices
The Internet of Things(IoT) is expanding the range of data available for risk assessment. From smart home devices to connected cars, IoT technology provides real-time data that can be used to monitor and mitigate risks.
Blockchain Integration
Blockchain technology offers a secure and transparent way to manage insurance contracts and claims. By integrating blockchain with AI and ML, insurers can enhance data security and streamline processes.
Expansion of Parametric Insurance
Parametric insurance, which pays out claims based on predefined parameters rather than actual losses, is gaining traction. AI and ML can enhance the accuracy of these parameters and automate the payout process, making parametric insurance more efficient and appealing.
Case Studies
To illustrate the transformative impact of AI and ML in insurance, let’s examine a few real-world case studies.
Lemonade
Lemonade is an insurance company that uses AI to streamline the entire insurance process. By leveraging AI chatbots for customer interactions and ML algorithms for underwriting and claims processing, Lemonade can offer competitive rates and faster service. Their AI-driven approach has allowed them to disrupt the traditional insurance market and attract a large customer base.
Ping An Insurance
Ping An, one of China’s largest insurers, has heavily invested in AI and ML to enhance its operations. Their AI-powered health insurance platform uses ML to analyze medical records and provide personalized health management advice. This not only improves customer health outcomes but also reduces claim costs for the insurer.
Zurich Insurance Group
Zurich Insurance Group has implemented AI to detect and prevent fraud. Their AI system analyzes claims data to identify suspicious patterns, significantly reducing fraudulent claims. Additionally, Zurich uses AI to optimize their pricing models, ensuring competitive premiums while maintaining profitability.
The Role of Regulatory Bodies
As AI and ML become more prevalent in the insurance industry, regulatory bodies play a crucial role in overseeing their implementation.
Ensuring Fair Practices
Regulators must ensure that AI and ML algorithms do not lead to discriminatory practices. This involves setting standards for algorithmic transparency and fairness.
Protecting Consumer Data
Data protection regulations must be updated to address the new challenges posed by AI and ML. Insurers should be required to implement robust data security measures and provide transparency about how customer data is used.
Promoting Innovation
Regulators should strike a balance between protecting consumers and promoting innovation. This can be achieved through regulatory sandboxes that allow insurers to test new AI and ML applications in a controlled environment.
ALSO READ:
Mastering Marine Insurance: Your Ultimate Important Guide to Protecting Maritime Ventures in 2024
Event Cancellation Insurance: Essential Protection for Unforeseen Circumstances in 2024
FAQs on AI and Machine Learning in Insurance
What is AI in the context of insurance?
AI, or artificial intelligence, in insurance refers to the use of advanced computer systems and algorithms to mimic human intelligence, enabling insurers to analyze data, make decisions, and automate processes more efficiently and accurately.
How does machine learning differ from AI in insurance?
Machine learning (ML) is a subset of AI focused on developing algorithms that allow systems to learn from data and improve over time. In insurance, ML is used to predict risks, detect fraud, and optimize pricing by analyzing large datasets and identifying patterns.
How are AI and ML transforming risk assessment in insurance?
AI and ML enhance risk assessment by utilizing a broader range of data sources, including structured data (e.g., age, health history) and unstructured data (e.g., social media, IoT devices). This allows for more accurate and personalized risk profiles and predictive analytics.
Can AI help in detecting insurance fraud?
Yes, AI can significantly improve fraud detection. AI systems analyze claims data to identify unusual patterns and anomalies indicative of fraudulent activity. These systems can flag suspicious claims for further investigation, reducing losses and speeding up legitimate claims.
How do AI and ML improve the customer experience in insurance?
AI and ML enhance customer experience by providing personalized recommendations, automating routine inquiries through chatbots, and offering proactive risk management advice. This leads to faster service, tailored products, and better overall satisfaction.
What are the benefits of using AI in claims processing?
AI benefits claims processing by automating routine tasks, accurately assessing damage through image analysis, detecting fraud, and facilitating faster settlements. This reduces administrative costs and improves the efficiency of handling claims.
Are there any ethical concerns with using AI and ML in insurance?
Yes, there are ethical concerns, such as potential biases in AI algorithms that could lead to discriminatory practices. Ensuring fairness and transparency in AI models is essential to address these issues and maintain trust.
How do AI and ML contribute to cost savings for insurers?
AI and ML contribute to cost savings by improving risk assessment accuracy, reducing fraudulent claims, and automating administrative tasks. These efficiencies lower operational costs and allow insurers to offer more competitive premiums.
What challenges do insurers face when implementing AI and ML?
Challenges include ensuring data privacy and security, addressing ethical concerns related to algorithmic bias, integrating AI with legacy IT systems, and complying with regulatory requirements.
How do regulatory bodies oversee the use of AI in insurance?
Regulatory bodies ensure that AI and ML practices in insurance are fair and transparent, protect consumer data, and promote innovation. This may involve setting standards for algorithmic fairness, updating data protection regulations, and creating regulatory sandboxes for testing new technologies.
What is the role of IoT in AI-driven insurance?
The Internet of Things (IoT) provides real-time data from connected devices (e.g., smart home sensors, telematics in cars) that AI systems can analyze to assess risks more accurately and offer proactive risk management solutions.
What is parametric insurance, and how does AI enhance it?
Parametric insurance pays out claims based on predefined parameters (e.g., a specific weather event) rather than actual losses. AI enhances parametric insurance by improving the accuracy of these parameters and automating the payout process, making it more efficient.
Can AI help in personalized insurance offerings?
Yes, AI can analyze individual customer data to tailor insurance products to their specific needs, preferences, and risk profiles. This personalization leads to more relevant coverage options and better customer satisfaction.
How does AI improve operational efficiency in insurance companies?
AI improves operational efficiency by automating routine administrative tasks (e.g., data entry, policy renewals), optimizing workflows, and enabling faster decision-making processes. This allows insurers to focus on more strategic activities.
What future trends can we expect in AI-driven insurance?
Future trends include more advanced predictive modeling, increased use of IoT devices, integration of blockchain technology for secure and transparent transactions, and the expansion of parametric insurance products.
Future trends include more advanced predictive modeling, increased use of IoT devices, integration of blockchain technology for secure and transparent transactions, and the expansion of parametric insurance products.
By addressing these frequently asked questions, we provide a comprehensive overview of how AI and ML are transforming the insurance industry, highlighting the benefits, challenges, and future prospects.
Conclusion
The integration of artificial intelligence (AI) and machine learning (ML) into the insurance industry represents a transformative leap that promises to redefine traditional practices and set new standards for efficiency, accuracy, and customer satisfaction. This technological revolution is not merely a trend but a fundamental shift that is reshaping the landscape of insurance from the ground up.
Enhanced Precision and Personalization
At the heart of this transformation is the enhanced precision and personalization that AI and ML bring to risk assessment and underwriting. Traditional methods, which relied heavily on historical data and broad categorizations, are being supplanted by dynamic models that incorporate real-time data from diverse sources such as social media, wearable technology, and telematics. This allows for the creation of comprehensive risk profiles and predictive analytics that are far more accurate and individualized. The result is an underwriting process that is not only more precise but also capable of offering personalized premiums and coverage options tailored to the unique risk profiles of individual customers.
Streamlined and Efficient Claims Processing
AI and ML are also revolutionizing claims processing, turning what was once a slow and cumbersome process into a streamlined, efficient operation. Automated systems can now handle routine tasks such as initial claims assessment and damage evaluation through advanced image recognition technologies. This automation extends to fraud detection, where AI systems can identify suspicious patterns and anomalies that human assessors might miss. The outcome is a claims process that is faster, less prone to error, and more secure, leading to quicker settlements and greater customer satisfaction.
Superior Customer Experience
The improvements in operational efficiency are matched by significant enhancements in the customer experience. AI-driven personalization allows insurers to offer customized product recommendations and proactive risk management advice. AI-powered chatbots and virtual assistants provide 24/7 support, guiding customers through policy selections and claims procedures with ease. This level of responsiveness and customization fosters stronger relationships between insurers and their customers, driving loyalty and satisfaction.
Operational Efficiency and Cost Savings
The benefits of AI and ML extend to the operational side of insurance companies as well. By automating many administrative tasks, these technologies reduce the need for manual intervention and minimize the risk of human error. This not only increases operational efficiency but also frees up human resources to focus on more strategic initiatives. The cost savings from improved risk management, reduced fraud, and streamlined operations can be substantial, allowing insurers to offer more competitive premiums.
Navigating Challenges and Ethical Considerations
Despite the clear benefits, the adoption of AI and ML is not without its challenges. Issues related to data privacy, security, and ethical concerns about algorithmic bias must be carefully managed. Regulatory bodies play a crucial role in ensuring that these technologies are implemented fairly and transparently, protecting consumer rights while promoting innovation.
Future Prospects and Continuous Innovation
Looking ahead, the future of AI and ML in insurance is bright, with continuous advancements driving further innovations. Insurers are expected to leverage these technologies to develop new products, such as parametric insurance, which pays out claims based on predefined parameters rather than actual losses. The increasing use of Internet of Things (IoT) devices will provide even more data for real-time risk assessment, while blockchain technology promises to enhance data security and transparency.
Final Thoughts
In conclusion, the incorporation of AI and ML into the insurance industry is a game-changer, offering enhanced accuracy, efficiency, and customer-centric services. Insurers that embrace these technologies will be well-positioned to lead in a competitive market, delivering greater value to their customers and adapting swiftly to emerging risks. As the industry continues to evolve, the strategic implementation of AI and ML will be crucial in driving sustained growth, innovation, and resilience. The future of insurance is intelligent, and the journey has only just begun.